Robert Brandon Gramacy
Education
- Ph.D. Applied Mathematics & Statistics, University of California, Santa Cruz, December 2005, advised by Herbert K.H. Lee, Dissertation: Bayesian treed Gaussian process models
- M.Sc. Computer Science, University of California, Santa Cruz, April 2003, advised by Manfred K. Warmuth, Thesis: Adaptive Caching by Experts
- College Honors; 4.00 GPA
B.Sc. (Highest Honors) Computer Science, University of California, Santa Cruz, June 2001, Honors Thesis: Shortest Paths and Network Flow Algorithms for ESD Analysis B.A. (Honors) Mathematics, University of California, Santa Cruz, June 2001, Project: Combinatorial Optimization by Matchings
Awards & Honors
- Robert King Steel Faculty Fellow, 2014-2015
- Kemper Family Foundation Foundation Scholar; 2011-2012
- R in Finance best academic paper prize; 2011
- INQUIRE UK/Europe best paper prize, 1 of 2 winners; 2008
- Savage Award for best Ph.D. thesis in Bayesian applied methodology; 2006
- ASA Statistical Computing & Graphics student paper competition, 1 of 4 winners; 2005
- UCSC Applied Math & Stats nomination for UC Presidents Dissertation fellowship; 2005
- Huffman Prize, given to the top graduating UCSC Engineering student; 2001
- Chancellor’s Award, UC Santa Cruz; 2001
- Dean’s Award, UC Santa Cruz Baskin Engineering School; 2001
- UCSC Student Employee of the Year; 2000
Professional Memberships
- American Statistical Association (ASA)
- International Society for Bayesian Analysis (ISBA)
- Institute of Mathematical Statistics (IMS)
- Royal Statistical Society (RSS)
- Institute for Operations Research and the Management Sciences (INFORMS)
- Classification Society (CS)
- Society for Industrial and Applied Mathematics (SIAM)
- Stat 3504: Nonparametric Statistics, 2016-17
- Stat 6984: Response Surface Methods and Computer Experiments, 2016-17
- BUS 41913: Bayesian Inference, Spring 2015-16
- BUS 41100: Applied Regression Analysis, Spring 2015-16
I am a computational statistician. I specialize in areas of real-data analysis where the ideal modeling apparatus is impractical, or where the current solutions are inefficient and thus skimp on fidelity. Such endeavors often require new models, new methods, and new algorithms. My goal is to be impactful in all three areas while remaining grounded in the needs of a motivating application. I aim to release general purpose software for consumption by the scientific community at large, not only other statisticians.
One example comes from my Ph.D. work, where I helped NASA design a computer experiment for a re-usable rocket booster. The software developed for this project, tgp for R, has since found wide applicability in areas as diverse as insurance, economics, climate science, epidemiology, and finance. My dissertation won three awards including the Savage Award for best thesis in applied Bayesian methodology.
Fast-forward to the present: I am excited about a new R package called laGP which is aimed at big data regression, and computer model emulation, by local approximate Gaussian processes. The code in the package, which facilitates massive parallelization, has been used to tackle a large-scale computer model calibration problem arising in a radiative shock hydrodynamics problem, and for blackbox constrained optimization in a benchmark groundwater remediation exercise. I am currently updating the methods for a cool application on predicting the atmospheric drag of satellites in orbit. This is important for positioning and collision detection. The University of Chicago Research Computing Center (RCC), to whom I am grateful for valuable high-performance computing (HPC) resources, did a puff piece on this work-in-progress.
I recently received NSF funding for a project blending optimal stochastic control, response surface modeling, and design of computer experiments. In early 2016 I recruited Mickaël Binois to join me as a postdoc, funded on the grant. We will work together to develop methods and computational tools for large scale heteroskedastic Gaussian process regression, as required by challenging dynamic control applications applications from finance, epidemiology, gas storage, and UAV tracking. Stay tuned.
For more information, please see my Research Page.
For a comprehensive list of my publications and technical reports, please visit my Publications Page.
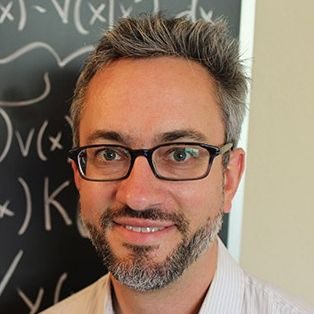
Professor
403G Hutcheson Hall (MC 0439)
250 Drillfield Dr.
Blacksburg, VA 24061